Summary: This article introduces A/B testing and split testing, highlighting their importance in gauging the success of websites and digital marketing campaigns. It also discusses critical differences, statistical methods, best practices, and considerations for accurate results.
Table of content:
1. Introduction to A/B Testing and Split Testing
2. Key Differences and Similarities Between A/B Testing and Split Testing
3. Statistical Methods for A/B Testing
4. Best Practices and Common Mistakes in A/B Testing
5. A/B Testing in Conversion Optimization
6. A/B Testing Variants and Considerations
Introduction to A/B Testing and Split Testing
A/B testing and split testing play a crucial role in digital marketing, acting as essential methods employed by advertisers and marketers to evaluate the performance of various online assets such as websites and email campaigns. For instance, in the context of a website, A/B testing entails comparing two different versions of a web page to determine which one yields better results, such as improved user engagement or higher conversion rates. On the other hand, split testing involves testing multiple variations of a webpage or app to ascertain which performs the best.
Furthermore, these testing methods are pivotal for making data-driven decisions to improve user experience and boost conversion rates. Using statistical tools, such as Fisher’s exact test, Pearson’s chi-squared test, Z-test, Student’s t-test, and Welch’s t-test, advertisers and marketers can quantitatively measure the impact of different elements or versions on user behavior and overall performance. This statistical analysis allows for identifying the most effective approach, helping to guide strategic decision-making in the digital marketing landscape.
Overall, A/B testing and split testing are indispensable tools that empower marketers and advertisers to optimize their online assets and marketing strategies, aiming for increased user engagement, higher conversion rates, and improved business outcomes.
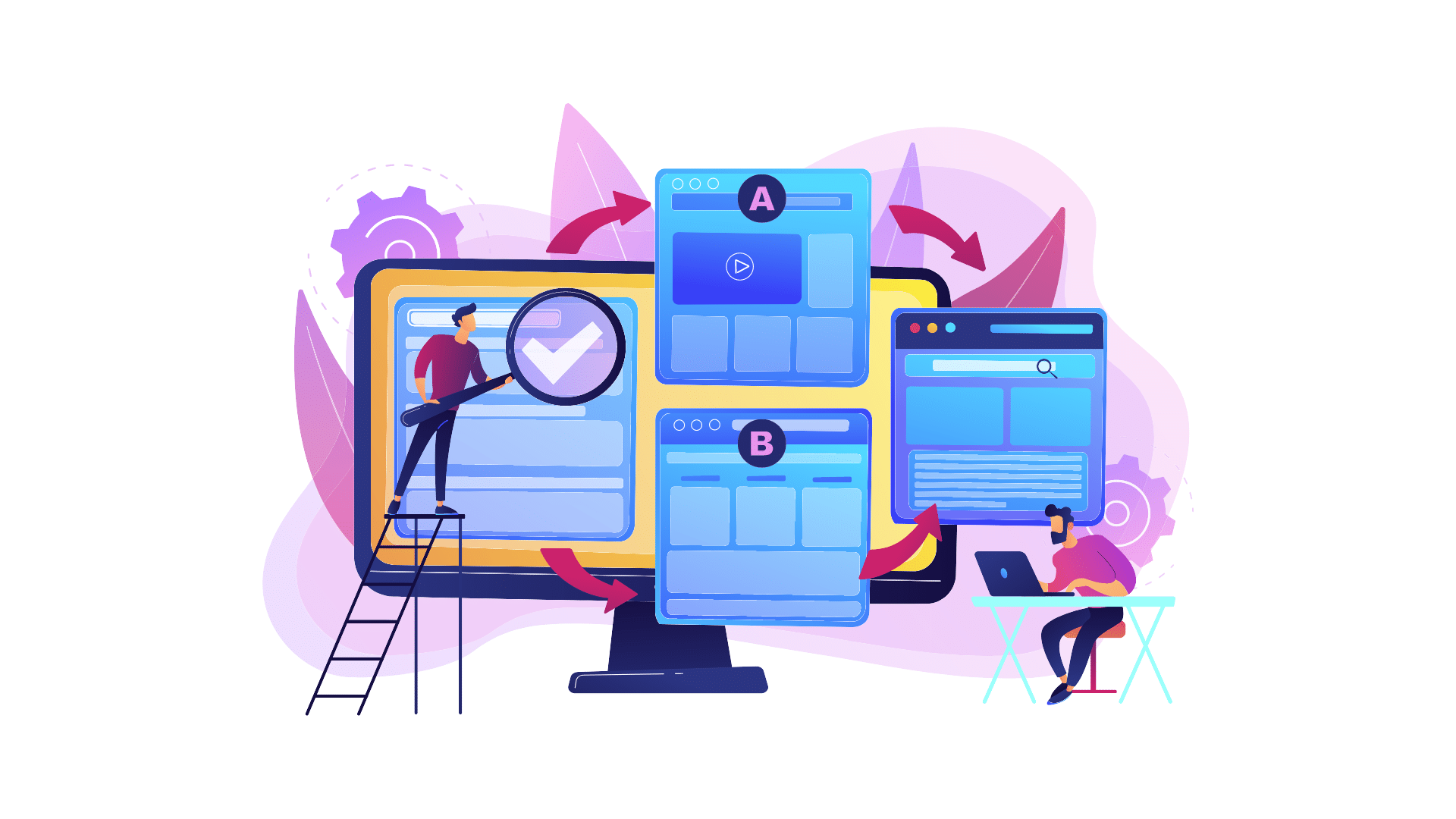
Key Differences and Similarities Between A/B Testing and Split Testing
A/B testing and split testing are essential methods used in digital marketing to assess the performance of different versions of websites, email campaigns, and other digital marketing elements. A/B testing involves comparing two versions of a webpage or app to determine which performs better, while split testing entails testing multiple variations to identify the most effective option.
One key difference between A/B testing and split testing lies in the scope of tested variations. A/B testing focuses on comparing two distinct versions, providing insights into the performance disparity between two specific iterations.
On the other hand, split testing allows for examining multiple variations, enabling marketers to understand the relative effectiveness of various elements within a single campaign or webpage.
Despite this difference, the ultimate goal of both A/B testing and split testing remains to increase conversions and user engagement. These testing methods are crucial for making data-driven decisions and improving the overall user experience within digital marketing initiatives. By leveraging statistical tools and rigorous experimentation, marketers can gain valuable insights into the performance of different elements and make informed decisions to enhance their digital marketing strategies and optimize their conversion rates.
Statistical Methods for A/B Testing
A fundamental aspect of A/B testing is using statistical methods to assess the performance of different website or digital marketing campaign versions. For discrete metrics like click-through rate and conversion rate, statistical tests such as Fisher’s exact test and Pearson’s chi-squared test are commonly employed to measure the effectiveness of different variations. For continuous metrics like average revenue per user and average session duration, statistical tests like the Z-test, Student’s t-test, and Welch’s t-test are utilized to make data-driven decisions based on the observed differences between the variants.
To illustrate, consider an e-commerce website conducting an A/B test to compare two versions of its product page. The website’s team may use a statistical method like the Z-test to determine if there is a significant difference in the average order value between the two versions. This statistical analysis enables the team to confidently identify which version is more effective in driving higher purchase values, leading to informed decision-making for future iterations of the product page.
Moreover, it is crucial to understand the significance of the p-value, which indicates the probability of observing a discrepancy between the samples. Based on the p-value, the statistical significance of the results is determined, guiding marketers and advertisers in making informed choices about which version to implement for optimal user experience and conversion rates.
Therefore, the careful selection and application of these statistical methods play a pivotal role in the success of A/B testing and ultimately contribute to enhancing digital marketing strategies.
Best Practices and Common Mistakes in A/B Testing
When conducting A/B testing, it’s crucial to avoid common mistakes that could undermine the accuracy and reliability of the results. One common pitfall is formulating an invalid hypothesis, which can lead to misguided conclusions and ineffective decision-making. For instance, if a hypothesis lacks clarity or fails to address a specific aspect of user behavior, the A/B test may not yield meaningful insights.
Additionally, testing too many components simultaneously can dilute the impact of individual changes, making it challenging to attribute any observed variations in results to specific alterations. Therefore, testing one element simultaneously is advisable to ensure precise and conclusive findings.
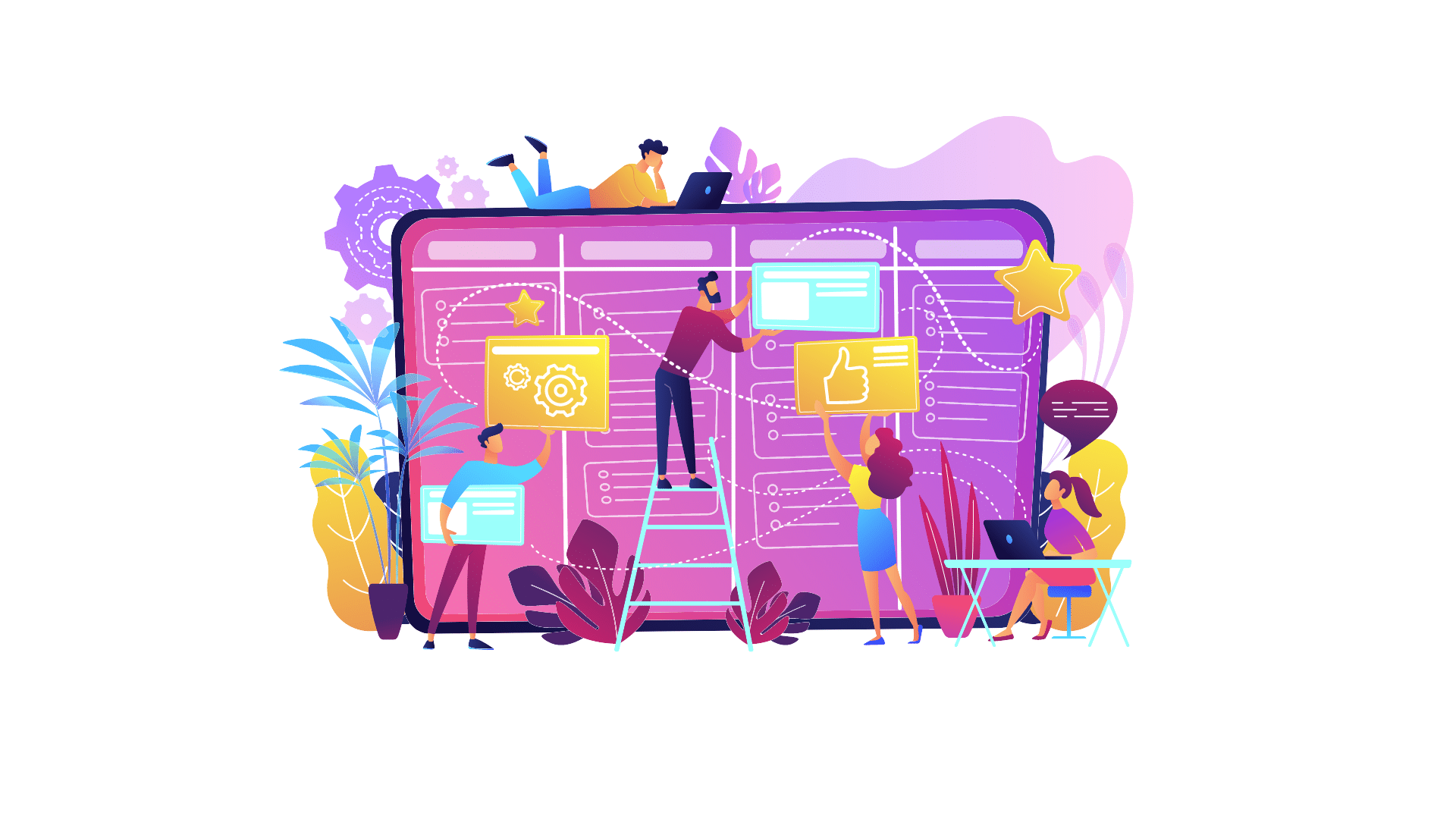
In contrast, adopting best practices can substantially optimize the A/B testing process. A structured approach to conversion optimization emphasizes the significance of meticulous planning and testing before the actual design phase of a website or marketing campaign. By defining the goal of the test and setting clear targets for the desired results, marketers can streamline the testing process and enhance the likelihood of achieving actionable insights.
Furthermore, prioritizing hypotheses based on their ease of implementation and potential impact can help identify the most crucial elements to test, thereby maximizing the efficiency and effectiveness of A/B testing initiatives. Overall, adopting best practices in A/B testing can elevate the quality of decision-making and contribute to more substantial improvements in conversion rates and user engagement.
A/B Testing in Conversion Optimization
A/B testing is an essential tool for quantifying changes in products and marketing plans, ultimately contributing to optimizing conversion rates and customer experience. It allows businesses to make data-driven decisions by testing incremental changes such as UX adjustments, new features, ranking, and page load times. For example, a company may use A/B testing to compare two landing page versions to determine which layout, design, or content leads to a higher conversion rate, optimizing the customer journey and user experience.
Furthermore, A/B testing is crucial for conversion rate optimization (CRO) as it helps identify and address potential barriers to conversion. By focusing on reducing fears, uncertainties, and doubts (FUDs), businesses can improve customer experience and increase conversion rates.
For instance, through A/B testing, organizations can test different call-to-action buttons, page copy, or promotional offers to determine which elements resonate better with their audience and drive higher conversion rates. This iterative testing and optimization process is fundamental to enhancing user experience and maximizing the impact of digital marketing efforts.
A/B Testing Variants and Considerations
A/B testing, or split testing, is widely used to compare two variants to determine which variable is more effective.
On the other hand, multivariate testing allows for comparing a higher number of variables and their interactions on a single page, making it suitable for advanced testers and large amounts of daily traffic. For example, if a digital marketing team wants to test different combinations of headlines, images, and calls-to-action on a website to determine the best-performing combination, multivariate testing would be the ideal approach due to the complexity of the variables involved.
Furthermore, when conducting A/B testing, it is essential to ensure that traffic allocation between the control and variation is evenly distributed to obtain accurate results. For instance, if one variation receives significantly more traffic than the other, the results may be skewed, leading to biased conclusions. In addition, integrating qualitative data, such as user feedback, surveys, and customer interviews, is essential to gain valuable insights into user behavior and preferences, ultimately contributing to a more comprehensive understanding of the test results. By incorporating qualitative data, businesses can avoid making decisions solely based on quantitative data and enhance their understanding of user needs and pain points, leading to more effective optimization strategies.
In summary, understanding the unique requirements of A/B testing variants and considering the importance of accurate results and qualitative data integration is crucial for successful testing and optimization in digital marketing. Each type of test offers distinct advantages and is uniquely suited to specific situations. By leveraging the appropriate test for the right circumstances, businesses can achieve the best return on investment for their testing campaigns.